MAI-SIM: structured illumination microscopy for the masses
Our Laser Analytics group hit the history books to develop a superresolution microscopy technique that will allow more research into the origin and treatment of diseases such as Alzheimer’s and cancer.
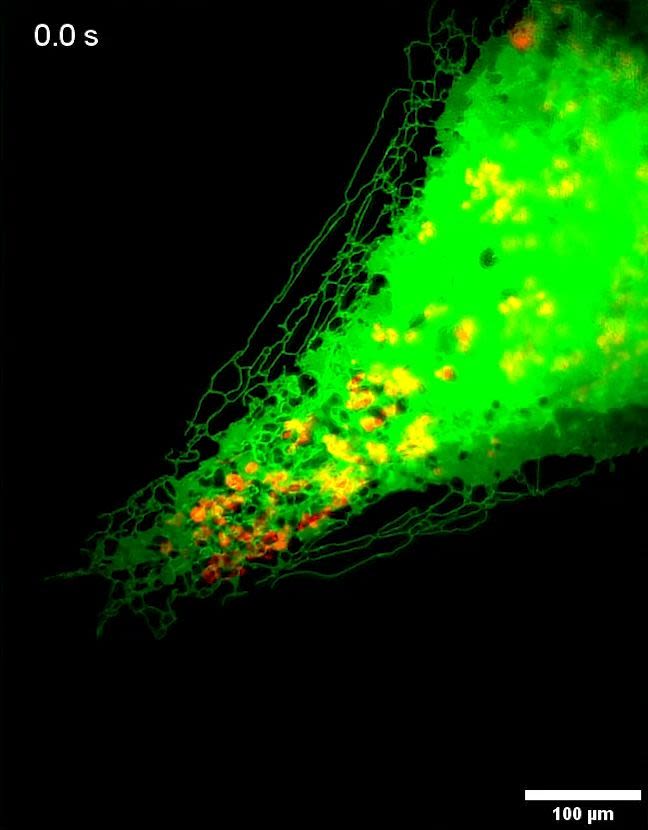
Scientists have been using microscopy to reveal the unseen machinations of life and the universe for centuries. As our understanding of the world around us has deepened, the need to see at an increasingly small scale has grown.
Our Laser Analytics group here at CEB is particularly interested in finding out how different organelles – the tiny machinery that keeps our cells alive and responsive – interact with one another, orchestrating the functions of life when we are healthy, or causing disease when things go wrong. These organelles, and their meticulously coordinated relationships, are so small and fleeting that they cannot be resolved with standard microscopes.
Researchers in the group, led by Professor Clemens Kaminski, use current understanding of physics, mathematics and computation to develop so-called ‘superresolution’ microscopy techniques, which enable them to see inside cells and track the movements of key organelles in real time.
They are particularly interested in processes that, when disrupted or hindered in some way, lead to the development of neurodegenerative disorders, such as Alzheimer’s and Parkinson’s disease.
Progress in treatment of these complex diseases requires the expertise and effort of a huge number of researchers. Yet much of the cutting-edge microscopy instrumentation required is prohibitively expensive. In their latest research, Kaminski and colleagues explain how they sought to make one of the most powerful tools in this context – structured illumination microscopy – available to as many biomedical researchers as possible, at a fraction of the usual cost. An endeavour that took them back to the very early days of microscopy.
Words by Professor Clemens Kaminski and Dr Edward Ward
The principle of structured illumination microscopy, SIM, is to create patterns of light that are projected onto a microscopic sample. The superposition of these light patterns with the patterns in the sample creates so called ‘beat patterns’ in the image, and these beat patterns convey information of detail in the sample that would be invisible when viewed through a normal microscope.
The physics of this can be easily understood because it is an effect that occurs in everyday life. For example, if you were to look through two layers of lace curtain from a distance, you would see such beat patterns appearing as stripes of lighter and darker shades, which change shape and direction as the curtains sway in a breeze. Although the pattern of the lace itself is too small to be seen by eye, the beat patterns are easily recognised.
Principle of structured illumination microscopy. The superposition of a sample pattern, here illustrated as a series of curved lines, with a striped illumination pattern leads to frequency beats, or Moiré fringes. These can be seen with low resolution instruments, even when you can’t see the original sample pattern.
Principle of structured illumination microscopy. The superposition of a sample pattern, here illustrated as a series of curved lines, with a striped illumination pattern leads to frequency beats, or Moiré fringes. These can be seen with low resolution instruments, even when you can’t see the original sample pattern.
In SIM we exploit this principle: we generate such beat patterns from microscopic objects and then calculate from the beat patterns what the underlying sample structure might be.
The principle is simple, and SIM microscopes are available commercially, but they have shortcomings. The first is that the traditional way of generating illumination patterns in SIM means that they only work optimally for one colour. To track the movements of multiple organelles at once, we need to ‘label’ them in different colours, so that we can distinguish one type of organelle from another. Traditional SIM microscopes use pixelated devices, such as those used in overhead projectors, to generate the illumination patterns, and with these it is not possible to generate the correct patterns for all of the colours you may be interested in.
The other problem lies in the very complex computation required to recover sample information from the SIM beat patterns, a process that is both time consuming and error prone.
To address these problems, we completely rethought how we would generate the light patterns used to illuminate the sample. We realised that we could use an instrument known as a Michelson interferometer to accomplish this.
The idea of using an interferometer to generate these patterns is fundamentally different to what was done before, and we had to go back to our physics textbooks to make the method work. It was a fascinating journey.
An historic breakthrough
The Michelson interferometer is one of the instruments most coveted by physicists, and it is closely tied to the history of physics itself. Originally designed by Albert Michelson in the 1880s it led to several breakthrough discoveries. It was used to obtain a precise measure of the speed of light in the famous Michelson-Morley experiment, which sought to prove the existence of ‘aether’ – a supposed medium permeating space that was thought to be the carrier of light waves – an idea that had to be abandoned after the experiment.
A schematic of Michelson’s original interferometer from his 1881 paper.
A schematic of Michelson’s original interferometer from his 1881 paper.
The Michelson interferometer is still used today in many different fields of research, including astronomy, spectroscopy, metrology, and materials testing. One of the most famous recent examples is the LIGO experiment for the detection of gravitational waves – accomplished by a gigantic Michelson interferometer. We are thus particularly pleased, that the instrument is now enabling a breakthrough in the field of microscopy.
The incredible beauty of this powerful instrument lies in its simplicity. In its essence, it consists of just three components: a glass plate to split a light beam in two, and two mirrors arranged in a perfect right angle to one another. These components can easily be seen in our design, which we have called machine learning assisted interferometric structured illumination microscopy (MAI-SIM).
Photograph of our MAI-SIM (machine learning assisted interferometric structured illumination microscopy) interferometer. The mirrors labelled 1, 2, 3 near the top end of the ruler take on the function of the mirrors labelled c and d, in Michelson’s original design in the previous figure.
Photograph of our MAI-SIM (machine learning assisted interferometric structured illumination microscopy) interferometer. The mirrors labelled 1, 2, 3 near the top end of the ruler take on the function of the mirrors labelled c and d, in Michelson’s original design in the previous figure.
In our instrument, two spatially separated, but otherwise identical, light beams are made to interfere in the sample. This generates so called Young’s interference fringes in the sample, exactly as those required for SIM microscopy.
The advantage of our method is that we can use the interferometer to very quickly step the illumination pattern through the sample and to change its orientation. Projecting different pattern orientations is essential for us to be able to probe all spatial details in the sample.
The beauty of the method is that stepping and reorientation of patterns can be achieved via simple rotation of a single mirror element in the interferometer. The other great advantage is that the Michelson interferometer works for any colour. We are thus able to project patterns of multiple colours into the sample simultaneously, greatly speeding up the method compared to alternatives. Furthermore, the patterns are always optimal, for any colour. Finally, mirror rotation can be accomplished at great speed, so our illumination patterns dance over the sample at frenetic pace.
The idea of producing Young’s fringes in the sample brings up another piece of physics history. The original experiment – the so-called Young’s double slit experiment – was also performed in the 19th century, by the physicist Thomas Young. He passed light through two narrow slits in a screen and observed that the light emerging behind it produces interference patterns, alternating bands of light and darkness. This experiment turned out to be one the most important ever devised. It was essential to support the wave theory of light and played an essential role in the conceptual development of quantum mechanics.
Thomas Young's sketch of two-slit diffraction of light. Narrow slits at A and B act as sources, and waves interfering in various phases are shown at C, D, E, and F. Young presented the results of this experiment to the Royal Society in 1803.
Thomas Young's sketch of two-slit diffraction of light. Narrow slits at A and B act as sources, and waves interfering in various phases are shown at C, D, E, and F. Young presented the results of this experiment to the Royal Society in 1803.
In our microscope the light emerging from the two slits is replaced by light beams produced in the Michelson interferometer.
Back to the future
We accompanied the development of the instrument with a machine learning algorithm that predicts the object’s structure from the rapidly produced SIM patterns. It is highly efficient computationally, and does not suffer some of the shortcomings of current methods.
In essence, we model the process of how SIM data is generated in our microscope with as much detail as possible. The trick then becomes to generate images that look as if they came from our microscope, but for which we know the structure of the underlying sample precisely, in order to train the algorithm to predict unknown structures. We used high resolution images of objects that are available on public image libraries and modelled what the SIM data would look like, if these objects were imaged through our microscope.
So, in essence we computed what beat patterns would result if we were to image the objects in our microscope, including noise from various sources that inevitably degrades any imaging experiment. We then fed this simulated data to a computer and ‘taught’ it how to interpret these to recover as much detail as possible from the original object. This method is called transfer learning and was spectacularly successful for our application; we were able to recover real SIM data with very good precision. Furthermore, the method is fast – so fast in fact, that we can reconstruct and view high resolution SIM data ‘live’, as it is recorded by the microscope.
A selection of our results can be seen below.
High resolution imaging in multiple colours
Top row: the resolution enhancement of MAI-SIM is seen when imaging small fluorescent beads of 200 nm (1 nanometre = 1 billionth of a metre) in size. In each panel, the top left-hand corner shows a conventional microscopy image, and the lower right shows the same sample when imaged with MAI-SIM. Going from left to right, the beads where imaged at different colours from blue to red. Middle row: Different organelles in the cell resolved at high resolution. Left: mitochondria; middle: endoplasmic reticulum; right: beta-tubulin. Bottom row: zoomed in regions demonstrating the resolution enhancement over conventional microscopy.
MAI-SIM resolves the intricacies of life at subcellular resolution
The top twelve panels give a fascinating insight into the interaction of lysosomes and the endoplasmic reticulum in the cell. Earlier research by the group demonstrated a dynamic ‘tug of war’ between the ER and lysosomes, with lysosomes dragging and pulling the ER into places where the cell requires it to be. MAI-SIM offers unprecedented detail on such interactions, including on where they go wrong, for example in the brain cells of patients suffering from neurodegenerative diseases.
Imaging multiple organelles simultaneously in living cells
The panels show a time sequence of moving mitochondria (shown in blue) and the endoplasmic reticulum, ER, (shown in magenta). One can clearly distinguish how the mitochondria, the energy factories of the cell, make dynamic contacts with the ER, which is the cell’s protein factory. The bottom row shows the resolution enhancement afforded by MAI-SIM (right panel) over conventional fluorescence microscopy.
Democratising superresolution techniques
Commercial SIM systems cost hundreds of thousands of pounds. Our MAI-SIM is more powerful than commercial systems, and is more than an order of magnitude cheaper to set up. We were particularly keen to document the setup and the software as meticulously as possible so that everybody can replicate it and benefit from its use.
The original working title of the paper was ‘MAI-SIM: interferometric multicolor structured illumination microscopy for everybody’ to reflect our desire to put state-of-the-art technology into the hand of as many researchers as possible – an effort to democratise superresolution microscopy.
Read the full paper, published in Nature Communications
Ward, E.N., Hecker, L., Christensen, C.N. et al. Machine learning assisted interferometric structured illumination microscopy for dynamic biological imaging. Nat Commun 13, 7836 (2022). https://doi.org/10.1038/s41467-022-35307-0
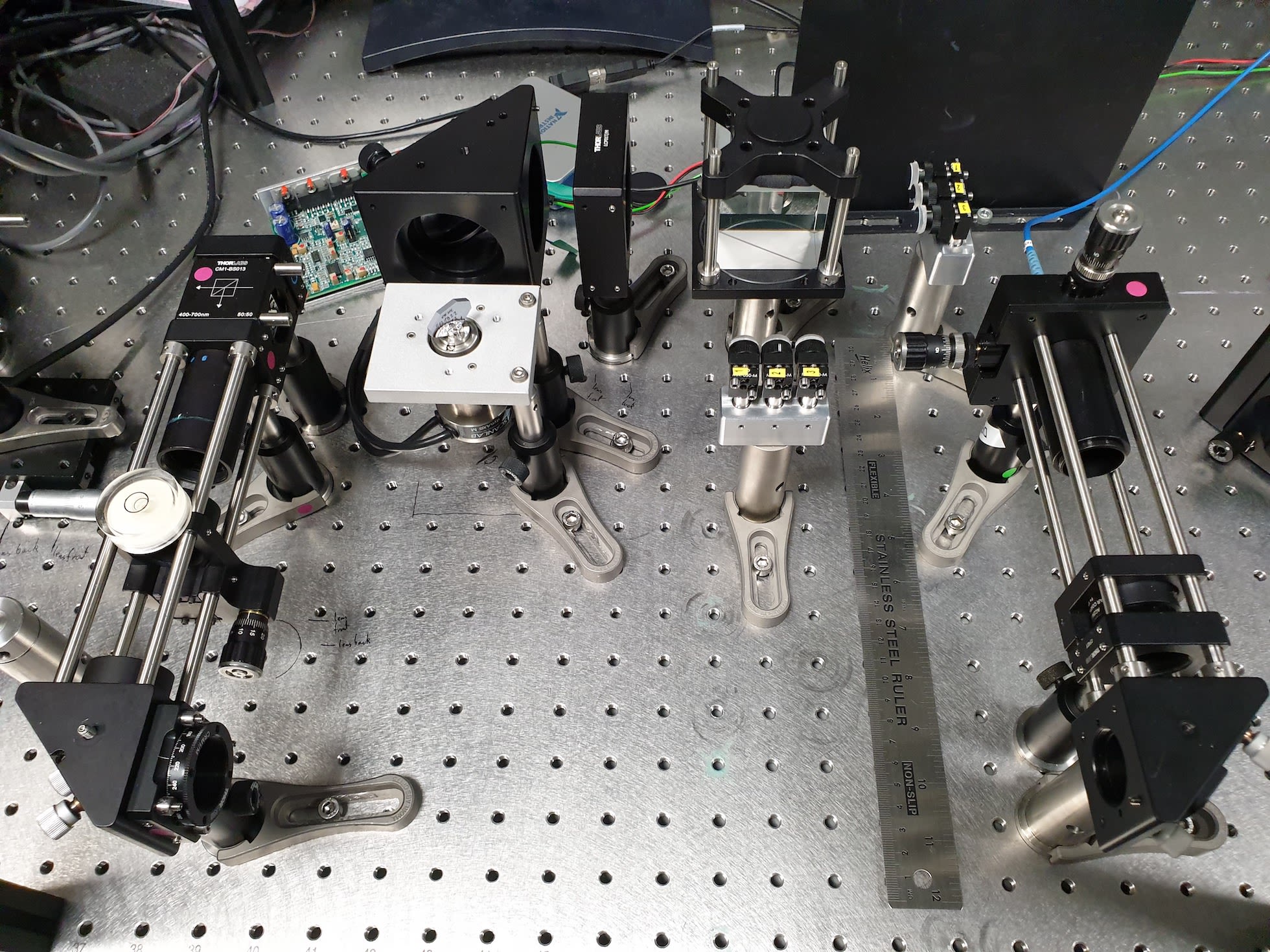